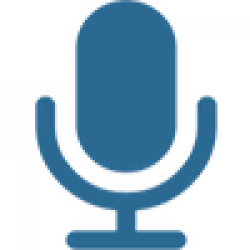
Micro-electrocorticography (μECoG) arrays are proposed as a minimally invasive alternatives to chronic intracortical microelectrode arrays. The promise of μECoG is to provide a neural interface with long lasting stability of both materials and recording ability. Since the surface potential typically lacks neuronal spikes, assessing recording quality is complicated by the question of what is being recorded, and from where. To address the these issues, we have developed simple but rigorous evaluation metrics of the sensitivity and specificity of μECoG signal to local neural processing in implanted arrays. To evaluate the detectability of stimulus-driven neural activity in a feature-free manner, we employ the Mahalanobis distance, which measures the statistical divergence of response from baseline. We also track the spatial random field of array recordings using a model to account for the share of variance from global from local processes, as well as from measurement noise. As a all-inclusive test of specificity and sensitivity, we track the accuracy and error of auditory stimulus decoding based on the driven neural response. As researchers continue to develop μECoG arrays for stable chronic neural interfaces, it is important to also demonstrate rigorous statistics of the recording abilities of new devices. We have developed straightforward and generalizable methods to evaluate μECoG recordings that we are using in conjunction with chronic reliability studies of novel arrays. While these methods are adapted in our lab to rodent auditory electrophysiology, they are intended to apply to array recordings in other models and sensory-motor systems.