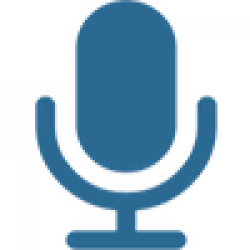
One of the paramount challenges of this century is that of understanding complex, dynamic, large-scale networks. Such high-dimensional networks, including social, financial, and biological networks, cover the planet and dominate modern life. In this talk, we propose novel approaches to inference in such networks, for both active (interventional) and passive (observational) learning scenarios. We highlight how timing could be utilized as a degree of freedom that provides rich information about the dynamics. This information allows resolving direction of causation even when only a subset of the nodes is observed (latent setting). In the presence of large data, we propose algorithms that identify optimal or near-optimal approximations to the topology of the network.