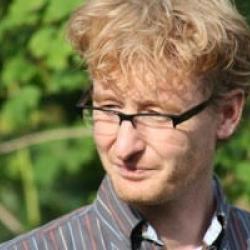
Increasingly deep learning applications are moving to the edge. Running high demand AI applications on small form factors puts very strict constraints on, among others, power efficiency of the underlying machine learning algorithms. We are thus witnessing a flurry of research activity on new ways to squeeze as much predictive power (a.k.a. intelligence) out of every Joule of available energy. For deep learning, one way to achieve this is to quantize the weights and activations of a neural network to the minimal possible number of bits required to make accurate predictions, sometimes even a single bit. However, learning with highly quantized weights and activations is very difficult due to the fact that gradients do not exist or are poorly approximated. Also, post-hoc quantization leads to a very high loss in accuracy. I will discuss a new way to achieve “quantization aware deep learning”. This means that we train the network in the cloud using high precision compute, but in such a way that quantization after training will lead to very small loss in accuracy. Our hammer is probabilistic deep learning which uses the probability of choosing a particular discrete value as a differentiable quantity amenable to back-propagation. We also include terms that encourage the weights and activations to cluster around the allowed (quantized) values. Experiments show that our method allows one to train highly quantized models without much loss in accuracy and improves on the current state of the art on this task.