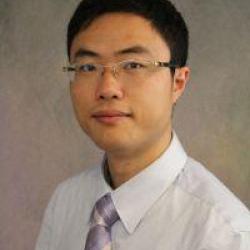
The Plug-and-Play (PnP) ADMM algorithm is a recently developed image restoration method that allows advanced image denoisers to be integrated into physical forward models to yield a provable convergent algorithm. Since its introduction in 2013, PnP ADMM has enabled numerous record-breaking image recovery results in deblurring, inpainting, super-resolution, Poisson denoising, and compressed sensing, etc. However, despite the successful applications and promising results, very little is known about why PnP ADMM performs so well. Fundamentally, the challenge lies in the fact that many of the latest denoisers are not easily expressible as proximal maps, e.g., deep neural networks. In this talk, I will highlight a few recent progresses made by my group and collaborators. I will discuss three questions. (1) Convergence: Under what conditions of the denoisers will PnP ADMM converge? Answering this question will allow us to comment on the kinds of denoisers we can use and the kinds of convergence we should expect. (2) Objective Function: By plugging in an off-the-shelf denoiser, what does PnP ADMM actually solve? That is, what is the corresponding objective function? This problem will tell us why and when PnP ADMM will perform well, and when PnP ADMM will fail. (3) Generalization: Are we able to generalize PnP ADMM to accommodate multiple agents beyond a single forward model and a single denoiser? This leads to a new concept called consensus equilibrium, which allows us to integrate multiple weak experts to produce an overall strong recovery method. I will illustrate the ideas through examples in image denoising, graph signal processing, turbulence removal and automatic foreground extraction.
Tel: (858) 822-4697
<tspackman@eng.ucsd.edu>