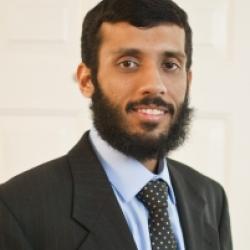
The theory of learning in games emerged to understand how repeated interactions among independent individuals can lead to certain equilibrium behaviors in the long run. Stochastic stability is a popular solution concept to explain the long term behavior for a class of learning dynamics called stochastic learning dynamics. In this talk, I will first establish that stochastic stability may not be a complete solution concept for stochastic learning dynamics. Then, I will motivate the need for comparing system behaviors under different stochastic learning rules at short, medium, and long time scales. Finally, I will present a novel framework for the comparative analysis of stochastic learning dynamics at different time scales. To highlight the insights that we can develop based on the proposed framework, I will compare two important learning dynamics namely Log-Linear Learning and Metropolis Learning.
<whughes@eng.ucsd.edu>