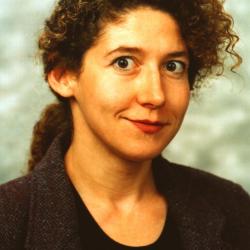
In many fields today, multiple sets of data are readily available. These might either be multimodal data where information about a given phenomenon is obtained through different types of acquisition techniques resulting in datasets with complementary information but essentially of different types, or multiset data where the datasets are all of the same type but acquired from different samples, at different time points, or under different conditions. Joint analysis of such data---its fusion---promises a more comprehensive and informative view of the task at hand, and, is at the heart of a multitude of problems such as those in neuroscience, remote sensing, computational social science, video analysis, atmospheric and physical sciences to name a few. Since, most often, very little prior information is available about the relationship among the datasets, data-driven methods based on matrix and tensor decompositions have proven especially useful. These solutions minimize the assumptions, and at the same time, can maximally exploit the interactions within and across the datasets. This talk presents an overview of the main models that have been developed and successfully used for fusion of multiple datasets. An important focus in the talk is on the interrelated concepts of uniqueness, interpretability, and diversity, which play a key role for data fusion. Diversity refers to any structural, numerical, or statistical property or assumption on the data that enables uniqueness, which is key for interpretability, the ability to attach a physical meaning to the final decomposition. The relevance of these concepts are highlighted through multiple examples, and the main challenges and the opportunities in the area are also addressed.
<bacarson@eng.ucsd.edu>