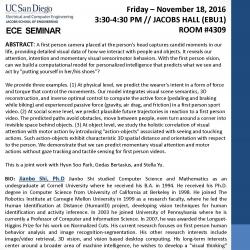
A first person camera placed at the person's head captures candid moments in our life, providing detailed visual data of how we interact with people and objects. It reveals our attention, intention and momentary visual sensorimotor behaviors. With the first person vision, can we build a computational model for personalized intelligence that predicts what we see and act by "putting yourself in her/his shoes"?
We provide three examples. (1) At physical level, we predict the wearer's intent in a form of force and torque that control the movements. Our model integrates visual scene semantics, 3D reconstruction, and inverse optimal control to compute the active force (pedaling and braking while biking) and experienced passive force (gravity, air drag, and friction) in a first person sport video. (2) At social scene level, we predict plausible future trajectories in reaction to a first person video. The predicted paths avoid obstacles, move between people, even turn around a corner into invisible space behind objects. (3) At object level, we study the holistic correlation of visual attention with motor action by introducing "action-objects" associated with seeing and touching actions. Such action-objects exhibit characteristic 3D spatial distance and orientation with respect to the person. We demonstrate that we can predict momentary visual attention and motor actions without gaze tracking and tactile sensing for first person videos.
This is a joint work with Hyun Soo Park, Gedas Bertasius, and Stella Yu.
(jmoritz@eng.ucsd.edu)