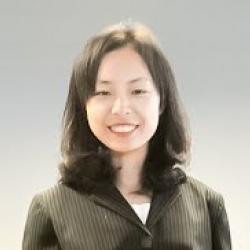
In this talk, I present three distinct problems in information theory, in which embedding appropriate structures plays a key role in finding optimal solutions. First, I propose a universal polarization technique, which alleviates the limitation of Arikan's channel-specific polar code design. Next, I describe how to design binary sequences such that the location of any length-$k$ chunk in the sequence can be uniquely determined via a noisy observation of the chunk. Such sequences can be used for phase detection in positioning systems. After that, I introduce a notion called graph information ratio, which unifies Shannon capacity of a graph and fractional chromatic number. A distance measure can be defined via graph information ratio, which characterizes the similarity between graphs. Finally, I conclude by briefly discussing future research directions in emerging data science applications through the lens of structure and complexity.