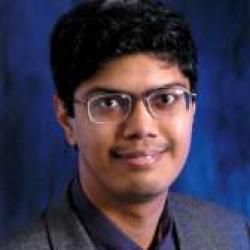
This lecture presents some of the prominent barriers to designing high performance and energy-efficient microprocessors and digital systems-on-chip in the sub-10nm technology regime and outlines new paradigm shifts necessary in next-generation tera-scale multi-core microprocessors and systems-on-chip. Emerging trends in SoC design for Artificial Intelligence, Machine Learning, and IoT platforms will be discussed, and key challenges in sub-10nm design are outlined, including (i) device and on-chip interconnect technology scaling projections, (ii) performance, leakage and voltage scalability, (iii) special-purpose hardware accelerators and reconfigurable co-processors for compute-intensive signal processing algorithms, (iv) fine-grain power management with integrated voltage regulators, and (v) resilient circuit design to enable robust variation-tolerant operation. Energy-efficient arithmetic and logic circuit techniques, static/dynamic supply scaling, on-die interconnect fabric circuits, ultra-low-voltage and near-threshold logic and memory circuit techniques, and multi-supply/multi-clock domain design for switching and leakage energy reduction are described. Special purpose hardware accelerators and data-path building blocks for enabling high GOPS/Watt on specialized DSP tasks such as machine learning, encryption, graphics and video/media processing are presented. Power efficient optimization of microprocessors to span a wide operating range across high performance servers to ultra-mobile SoCs, dynamic on-the fly reconfigurability and adaptation, and circuit techniques for active/standby-mode leakage reduction with robust low-voltage operability are reviewed. Specific chip design examples and case studies supported by silicon measurements and trade-offs will be discussed. Finally, emerging industry trends in neuromorphic computing will be outlined.
bacarson@eng.ucsd.edu
Phone: 858-822-6347