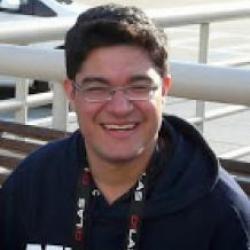
Generative Adversarial Network (GAN) is a powerful idea to train generative models and has recently shown amazing results in computer vision. Broadly, the framework estimates generative models via an adversarial process in which the generator and discriminator play a two-player game. Although there are only a few successful cases, the GAN framework is useful for NLP tasks and overcomes the inherent limitations in conventional methods. In this talk, we will first discuss a game-theoretic formulation of the GAN architecture and evaluate the framework in an image generation task. Next, we will address the limitations of our vanilla framework and study modifications that circumvent common training problems including mode collapse and non-convergence. Equipped with this knowledge, we extend the GAN framework to natural language and review techniques that enable training with discrete outputs. Subsequently, we introduce adversarial training and study its commonly used configurations including domain adaptation. Finally, we demonstrate applications of GAN in building conversational models, neural translation models, text style transformations and related NLP tasks.
dbharadi@ucsd.edu