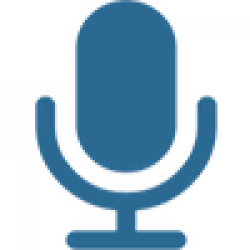
Universal coding, prediction and learning consider the situation where the data generating mechanism is unknown or non-existent, and the goal of the universal predictor/learner is to compete with the best hypothesis from a given hypothesis class, either on the average or in the worst-case. Multiple universality considers the case where the relevant hypothesis class is also unknown. In addressing large model classes, it will be useful to present the large hypothesis class as a union of smaller classes, possibly with different complexities, and the goal is not only to compete with the best hypothesis but also to identify the relevant hypothesis class with respect to which the competition is done. A main challenge is to correctly define the universality criterion so that the extra “regret” for not knowing the relevant class is monitored. We propose several possible definitions and derive their min-max optimal solutions, including the suggestion of an hierarchy of such sets. Interestingly, the proposed approach can be used to obtain Elias codes for universal representation of the integers, as a canonical example. Further, we explicitly present the multiple universality approach for general linear models, including linear regression, logistic regression and Perceptron. Finally, we show (including some empirical evidence) how multiple universality, with its non-uniform convergence and regret bounds, can be applied to explain and design learning schemes for general, large, even huge, “over-parameterized” model classes such as deep neural networks, transformers and so on.about none other that large language models (caveat: Information theoretic perspective).
Parallel to his academic career, he is closely involved with the high-tech industry: he founded 5 companies, among them Peach Networks (Acq: MSFT) and Amimon (Acq:LON.VTC). Recently, with his renewed interest in machine learning and AI, he co-founded Run:ai, a virtualization, orchestration, and acceleration platform for AI infrastructure.
Prof. Feder received several academic and professional awards among them the “creative thinking” award of the IDF, the Research Prize of the Israeli Electronic Industry, awarded by the President of Israel, the IEEE Information Theory Society best paper award and Information Theory Society Padovani lecturer. For the technology he developed in Amimon, he received the 2020 Scientific and Engineering Award of the Academy of Motion Picture Arts and Sciences (OSCAR) and was announced the principal inventor of the technology that attained the 73rd Engineering Emmy Award of the Television Academy.