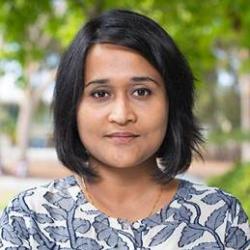
Compressive sensing has emerged as a central area of research in modern signal processing with applications in many scientific and engineering problems across disciplines. The key idea is to exploit the sparse representation of signals in appropriate transform domains, which allows exact and stable reconstruction of high dimensional signals from measurements of size proportional to the sparsity level. However, for many statistical signal processing problems that involve parameter estimation, the assumption of sparsity over a finite dictionary is somewhat artificially imposed by discretizing a continuous parameter space, which leads to undesirable grid-mismatch effects. Furthermore, it may be an overkill to reconstruct the signals itself, since the end-goal is to perform statistical inference. In this talk, I will demonstrate the possibility of compressively sampling parametric random processes, without requiring the underlying process to exhibit conventional notions of sparsity. What enables compression in this case is clever exploitation of the statistical priors on the unknown signals via design of highly structured deterministic samplers. The main ideas will be demonstrated by studying the problem of line spectrum estimation in temporal and spatial domains. Besides discussing sampling geometries and robust reconstruction algorithms based on both Maximum Likelihood criterion and super resolution subspace-based methods, I will also address fundamental performance limits of such compressive sampling schemes by deriving new expressions for Cramer-Rao bounds on the estimation error of line spectrum parameters. We will distinguish between conditional and unconditional Cramer-Rao bounds and argue that the latter is the appropriate fundamental bound in the compressive regime. The functional form of the Cramer-Rao bound will directly reveal the role of the sampling geometry and address important questions regarding its robustness.
Joint work with Ali Koochakzadeh and Heng Qiao.
Jacobs Hall, Room 2903
858-534-2498
clwills@eng.ucsd.edu