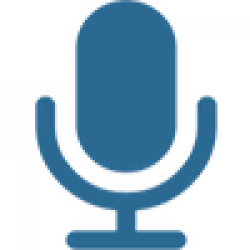
Within the wide field of sparse approximation, convolutional sparse coding (CSC) has gained increasing attention in recent years by assuming a global structured convolutional dictionary. While several works have been devoted to the practical aspects of this model, a systematic theoretical understanding of CSC seems to have been left aside. In this talk I will present a novel analysis of the CSC problem based on the observation that, while being global, this model can be characterized and analyzed locally. By imposing only local sparsity conditions, we show that uniqueness of solutions, stability to noise contamination and success of pursuit algorithms are globally guaranteed, resulting in much stronger and informative bounds. I will then present an extension of this model, the Multi-Layer CSC, and show its close relation to Convolutional Neural Networks (CNNs). This connection brings a fresh view to CNNs, as one can attribute to this architecture theoretical claims under local sparse assumptions, which shed light on ways of improving the design and implementation of these networks. We will further develop a sound pursuit for signals in this model by adopting a projection approach, providing bounds on the stability of its solution and analyzing different alternatives to implement this in practice. Last, but not least, we will derive a learning algorithm for the ML-CSC and demonstrate its applicability for several applications in an unsupervised setting.
Tspackman@ucsd.edu